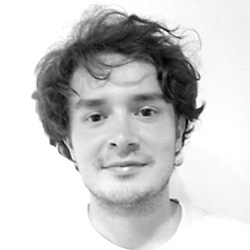
Edouard Oyallon, CNRS research fellow at ISIR, will be defending his habilitation to supervise research (HDR) on Friday 8 December at 2pm, at Sorbonne University’s Faculty of Science and Engineering.
Title of work: “Contributions to Local, Asynchronous and Decentralized Learning, and to Geometric Deep Learning“.
The composition of the jury is as follows:
- Jamal ATIF, Professor, University of Paris Dauphine-PSL (Examiner),
- Émilie CHOUZENOUX, Research Director, Inria (Reporter),
- Patrick GALLINARI, Professor, Sorbonne University/Criteo (Examiner),
- Julien MAIRAL, Research Director, Inria ( Reporter),
- Sebastian STICH, Researcher, CISPA ( Reporter),
- Michal VALKO, Researcher, Inria/Google Deepmind (Examiner).
Summary:
The research topics I will be discussing relate to the notions of distributed learning, local learning and deep representation learning on varieties. One of the central points that I am seeking to develop is the creation of a distributed deep neural network training algorithm that is efficient in terms of both computation time and the amount of operation required for training. The first chapter provides an introduction to these fields and to my research activities. The second chapter discusses the relationships between computer hardware and existing training paradigms. These interactions are important because they allow us to promise or obtain significant improvements in the training costs of deep networks. The third chapter describes the initial elements of a search to create deep representations on variety and graph. In particular, we have tried to think of principles for modelling such architectures. The fourth chapter discusses the local learning results that I have obtained, and in particular layer-by-layer gluttonous learning. This corresponds to the idea of training networks by proposing updates to the weights of a layer based solely on information local to that layer, and using as little as possible of the global information linked to all the layers. The fifth chapter summarises results obtained in decentralised asynchronous learning, in convex optimisation and deep neural network weight optimisation frameworks. One of the interests of this type of approach is their potential superiority, both from a practical implementation point of view and from an algorithmic point of view. Finally, the last chapter proposes future perspectives on my research.
Contact: Edouard Oyallon, CNRS research fellow