A team of researchers from LTCI, Télécom Paris, Institut polytechnique de Paris and ISIR, Sorbonne University, CNRS receives the best paper award at the ACM ICMI 2021 conference in Montreal, Canada.
The team is composed of Lucien Maman (Télécom Paris), Laurence Likforman-Sulem (Télécom Paris), Giovanna Varni (Télécom Paris) and Mohamed Chetouani (ISIR, Sorbonne University).
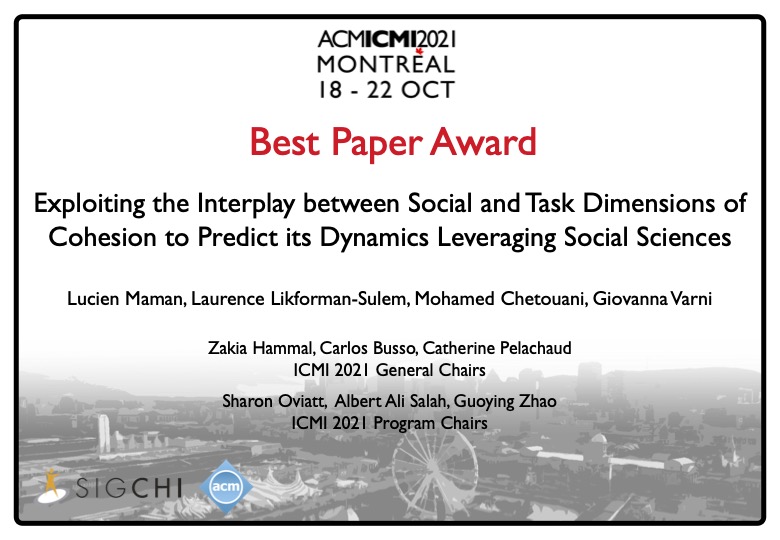
The paper entitled “Exploiting the Interplay between Social and Task Dimensions of Cohesion to Predict its Dynamics Leveraging Social Sciences” was produced in the framework of the ANR JCJC GRACE project (ANR-18-CE33-0003-01) led by Giovanna Varni and funded under the Artificial Intelligence Plan.
The ACM ICMI 2021 conference, held in Hybrid from 18 to 22 October 2021, is the first international forum for multidisciplinary research on multimodal human-human and human-machine interaction, interfaces and system development. The conference addresses the theoretical and empirical foundations, technologies and techniques of multimodal processing that define the field of multimodal interaction analysis, interface design and system development.
Emergent states are behavioural, cognitive and affective processes that emerge among group members when they interact together. Over the past decade, the development of computational approaches has led to a growing interest in building human-centred systems. Such development is particularly challenging because some of these states have multiple dimensions that interact in some way over time.
In this article we focus on cohesion, its dimensions and their interaction over time. There are several definitions of cohesion, it can be defined simply as the tendency of a group to stick together to pursue goals and/or emotional needs. This abundance of definitions has led to many different dimensions of cohesion. The “Social” and “Task” dimensions are the most studied, both in the social sciences and in computer science, as they both play an important role in a wide range of contexts and groups. However, to our knowledge, no previous work on predicting cohesion dynamics has focused on how these two dimensions interact. We draw on the Social Sciences to address this issue. In particular, we take advantage of the importance of Social cohesion to create flexible and constructive relationships in order to strengthen the Task dimension. We describe a deep learning architecture for predicting the dynamics of the Task dimension by applying transfer learning from a pre-trained model dedicated to predicting the dynamics of Social cohesion. Our architecture is evaluated against several baseline models. The results show that it significantly improves the predictions of the dynamics of the Task dimension, confirming the benefits of integrating social science knowledge into computational models.
Contact: Mohamed Chetouani, University Professor