The advent of artificial intelligences and robots is inducing profound transformations in our societies. Isir researchers help to anticipate them by working on the autonomy of machines and their ability to interact with human beings.
Gathered in multidisciplinary teams, they create drones, micro-tweezers, bionic prostheses, social robots, surgical arms and all kinds of intelligent and interactive systems, physical, virtual or mixed reality. Their applications address major societal challenges: health, industry of the future, transport, personal services.
Our research
We develop models from these:
Engineering, to characterize the dynamic behavior of high mobility robots in complex physical interaction with their environment.
Neurosciences or human movement sciences, to describe the cognitive and sensory-motor functions of living beings and to exploit them in the control of robots.
Psychology, for the characterization of the socio-interactive behaviors of human beings, among themselves or with machines.
We design and produce:
Robotic systems, real or simulated, equipped with algorithms for the control and processing of information, to achieve sensorimotor, learning, or interaction capacities superior to those of the state of the art.
Interfaces, to optimize the interaction between people and their environments, digital, physical, or mixed.
We validate these proposals:
Through experiments which realistically reproduce the environment of the targeted applications, in order to verify their robustness (for example: health, factory of the future, all-terrain mobility).
Through experimental campaigns involving human people. For this, we define rigorous protocols to guarantee the scientific value of the results, in compliance with ethical rules and laws protecting the person.
Institutions
ISIR is under the dual supervision of Sorbonne University, which is a world-class multidisciplinary university, and The French National Centre for Scientific Research (CNRS), which is one of the most important research institutions in the world.
The National Institute for Health and Medical Research (Inserm) is also the supervisor of the AGATHE team for its medical research.
Because of these three institutions, ISIR is attached to:
– For Sorbonne University, to the UFR919 of engineering under the Faculty of Science and Engineering.
– For the CNRS, it is attached primarily to the Institute CNRS Informatics, and secondarily to the Institute CNRS Biology, and the Institute CNRS Engineering.
– For Inserm, the AGATHE team is attached to the Inserm Institute of Technologies for Health.
Valuation and transfert
Partnership research
The ISIR is involved in numerous collaborations with companies. We support our partners to strengthen their innovation capabilities and gain competitiveness on new markets opened by robotics and artificial intelligence in many sectors.
Our partners
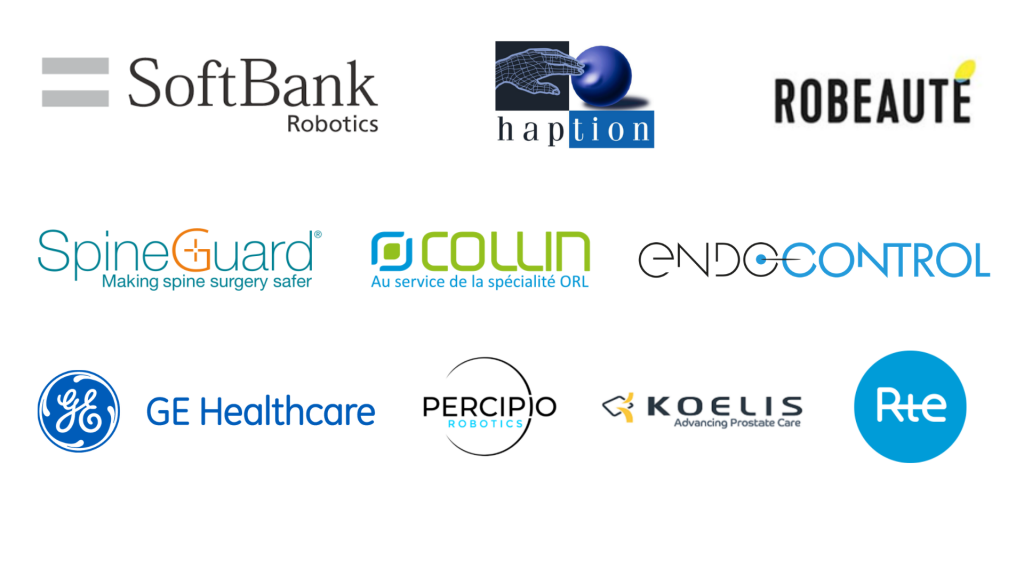
Start-ups
ISIR researchers are also at the origin of several start-ups, either because they are personally involved in their operation, or because they transfer technologies through the assignment of rights to exploit inventions or know-how.
Start-ups resulting from the Isir
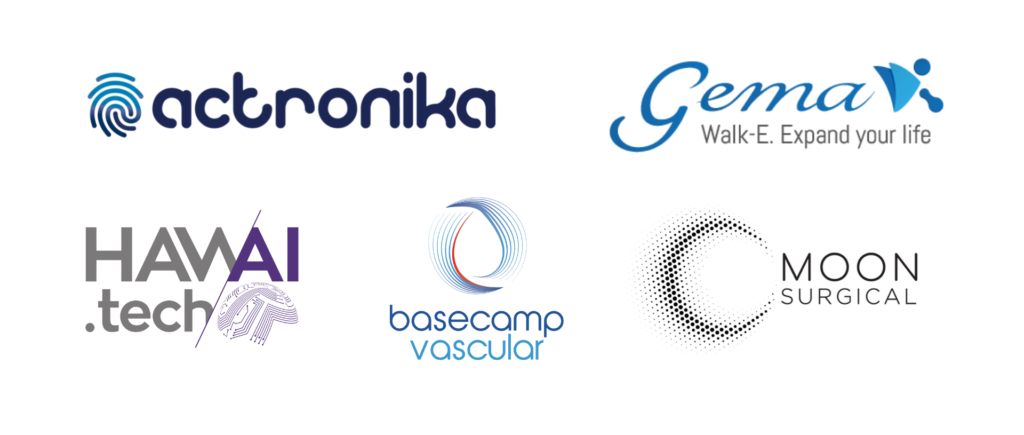
Equipment and platforms
With more than 800 m² dedicated to experimental activities, the ISIR maintains one of the largest robot parks in Europe.
The experimental platforms support the activities of all teams. They are made up of commercially available devices, or made by the technical department in our workshops. You wish to use them for your R&D studies? It is possible, contact us.
Three platforms support federating projects selected by its Scientific Council and supported via shared resources at the ISIR:
– GRETA, a virtual avatar equipped with multimodal social interaction capabilities,
– CoVR, a unique combination of motion tracking and analysis, virtual or augmented reality, and the movement of the environment or the subject placed in immersion,
– Surgical Cockpit, a set of interfaces for assisting gestures in minimally invasive surgery.
Other platforms:
– The Social Robotics Platform, which brings together, on the Jussieu campus and on the hospital sites of the Pitié Salpêtrière and Charles Foix in Ivry, many resources for the implementation and evaluation of social interaction mechanisms,
– The micro-robotics platform which integrates means of manipulation on microscopic scales and control interface with haptic feedback,
– The all-terrain robotics platform and its aerial, terrestrial or marine drones,
– The handling robotics platform, with single or double-armed handling devices covering a wide range of technologies.
Our 6 teams
Understanding how animals, including humans, perceive the world, decide what to do, learn from their mistakes and successes, interact with each other and with their environment: these fundamental questions are at the heart of the ACIDE team’s research activity. They also feed into work aimed at improving human-machine interfaces (computers, conversational agents, virtual reality systems, robots).
The ASIMOV team works on issues related to the interaction of robots with their environment. The areas of research concern the control of complex mechanical systems, endowed with action and perception capabilities, and subject to physical interactions with unknown and dynamic environments. The ultimate aim is to meet the challenges of service robotics in open environments.
MLIA is an Artificial Intelligence research team focused on designing statistical learning algorithms. The research is primarily aimed at developing deep learning applications, especially those concerning machine learning for robotics, vision and language, user interactions, and the modeling of complex systems in physics.
The team brings together researchers in robotics, computer science, neuroscience and health, all of whom share the aim of developing devices capable of interacting with humans in all their variety, in an intuitive and synergistic way. The variety of human beings implies the ability of devices to adapt to specific situations (ageing, disability, work, etc.). Intuitiveness and synergy presuppose a better understanding of how humans perceive and understand interaction with devices (objects, computers, robots), which is why we are exploring the fields of haptics, neuroscience, motor control and computational control and modelling to gain a better understanding of these sensorimotor interactions.
The RPI-Bio team is tackling a number of major scientific challenges, focusing on microrobotics, surgical robotics, multimodal perception, HMI, advanced control of robotic systems, machine and human learning and the transfer of expert skills (tele-expertise). The advances that will be made in these areas will undoubtedly provide the best possible response to the needs of tomorrow’s medicine and biology.