ACDC project – a french acronym for Counterfactual Learning for Controlled Data-to-text
The high availability of data is a well-established fact in our society. Whether the data comes from texts, user traces, sensors or knowledge bases, one of the common challenges is quickly access the information contained in these data. One of the answers to this challenge is to generate textual summaries of the considered data, as natural language has many advantages in terms of interpretability, compositionality, accessibility and transferability. The generation of textual descriptions is a problem that refers to an emerging field in the field of natural language processing, called Data-to-Text.
The ACDC project – a french acronym for Counterfactual Learning for Controlled Data-to-text – is an ANR project that builds on recent advances in deep learning and language generation for the generation of textual summaries from tabular data. It focuses on :
- the invariance search of input data,
- the extraction of high-level selection/compression operators,
- and the personalization of the produced outputs.
Coordinated by Sylvain Lamprier, Researcher at ISIR and Lecturer in Computer Science at Sorbonne University, the ACDC consortium groups partners with strong competences in machine learning, data-to-text, information retrieval and natural language generation. It is composed of the MLIA team of ISIR, the start-up reciTAL, the Laboratory of Analysis and Modeling of Systems for Decision Support (Paris Dauphine University), and finally the National Museum of Natural History (MNHN – Sorbonne University) which brings its scientific expertise in the field of biology which is one of the important case studies considered”.
Description of the ACDC project by Sylvain Lamprier, project coordinator.
What is the project about?
The ACDC project – Counterfactual Learning for Controlled Data-to-text – relies on advances in language generation via neural architectures, to address problems of textual synthesis of information contained in tabular data. We focus mainly on the search for invariance in the input data, the extraction of high-level compression operators and the personalization of the output produced. We propose to rely on deep learning and reinforcement techniques, involving inference, manipulation and decoding of content synthesis operations representations in a continuous semantic space.
What is the objective of the project?
All recent data-to-text approaches work in a supervised way, without explicit representation of the extraction operators they manipulate to go from global tabular content to textual synthesis.
The objective of the project is to produce regular representation spaces, encoding various types of semantic symmetry of the operators applied to the contents, allowing to control the compression mode of the generated texts, according to an input table. This project stands out because it proposes to focus on the expression of extraction operators, in order to gain in interpretability of the models, as well as in control capacity over the generated texts.
Our approach is therefore to try to deduce the content extraction operators allowing to go from a table to an observed text, with the aim of having a robust learning, which is both highly generalizable and controllable by a user. The challenges that this project targets are therefore: 1) the inference of information extraction operators in tables, 2) the management of heterogeneity in the input data and 3) the controlled synthesis of textual descriptions.
What are the possible applications?
Of course we don’t aim at reaching a human level to interpret data tables. Meanwhile, we are convinced that the methods we are considering will have a strong impact for the scientific community, because they define high-level adaptation mechanisms for data understanding, in the targeted application frameworks. The recent advances in deep learning (e.g. structural transformers), allow us to target this kind of objectives, which will constitute an important step for the community towards generalizable and customizable systems, whose learning doesn’t only imitate the observed outputs but seeks to combine complex extraction strategies to meet poorly defined needs.
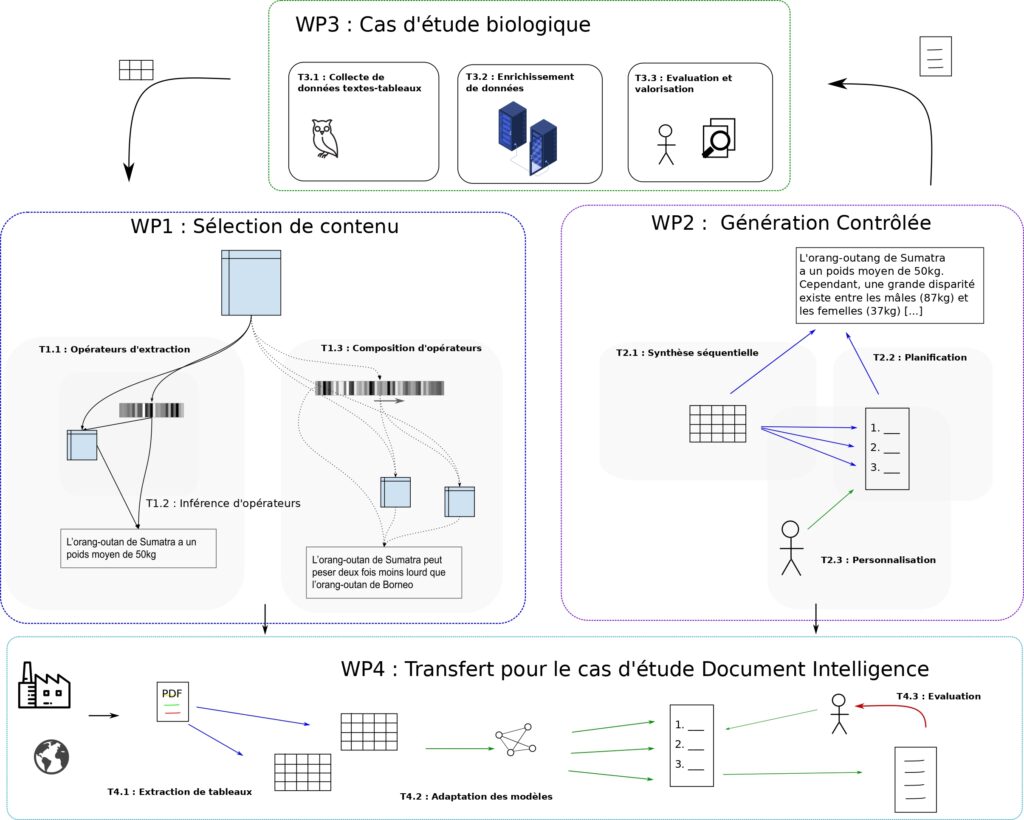
ACDC is a research project that is in line with the ISIR themes, by its language processing aspects, which make it part of the federative project Language and Semantics. The aim is to make machines speak, not only as speech machines on well-specified contents, but on salient aspects of input data, with selection of the important content and synthetic restitution. Also, because of its machine learning and reinforcement aspects, it fits into the themes of the Open-Ended Learning project. We address here issues around progressive learning, interpretability of manipulated representations, counter-factual learning and knowledge transfer. Its “Humans in the loop” aspects, aiming at the personalization of contents produced by interactions with users, also place it in a central position for the laboratory’s themes.
Contact for the project: Sylvain Lamprier, Researcher at ISIR