LOCOST : LOng COntext abstractive summarization with STate-space models
As the amount of textual data generated every day continues to increase exponentially, the need for tools capable of synthesising, analysing and understanding vast quantities of text has become essential in many fields. The state of the art, represented by neural architectures based on “transformers”, presents limitations in terms of resource consumption, especially when the texts to be processed are long.
The LOCOST model has emerged in this context of growing demand for automatic natural language processing. Its aim is to find a more resource-efficient method capable of processing texts of several dozen pages. LOCOST represents an innovative response to the challenges posed by the processing of long texts, opening up new prospects for the analysis and understanding of textual information on a large scale.
This work is being led by a team of researchers from various French and Singaporean institutions, including ISIR (CNRS/Sorbonne Université), LAMSADE at (CNRS/Université Paris Dauphine-PSL), the Criteo AI Lab, the Institute for Infocomm Research (A*Star), Nanyang Technological University, and AgroParisTech.
Description of the LOCOST model by ISIR members involved in this work.
Innovation compared with traditional word processing models
The main challenge of the LOCOST model is to find an efficient alternative to models that represent the state of the art in text generation, known as “transformers”. Although the latter are widely used for text generation, they consume considerable computing resources when confronted with large texts.
This is where LOCOST’s innovation comes in, using an encoder-decoder architecture based on state-space models with sub-quadratic complexity as a function of the model input. The research team succeeded in designing a system capable of handling text sequences of up to 500,000 words, while offering performance comparable to traditional “transformers”. However, what really sets this model apart is its efficiency: it saves up to 50% of memory during training and up to 87% during inference.
Towards new textual horizons
The applications of this discovery are vast. Not only does it pave the way for more efficient processing of long texts, but it also offers new perspectives for fields such as law, medicine and literature, which require the processing of large volumes of text.
The project members have already begun to explore various applications for their model, including the automatic summarisation of long texts such as scientific documents, administrative reports and books. But the possibilities don’t stop there. The team also plans to extend their architecture to other text generation tasks, such as the translation of long documents, as well as to other modalities such as the processing of spreadsheet files.
Although the state-space architecture used by LOCOST is still relatively new, the research team is optimistic about its potential for future improvement. They hope to refine their system further to make it even more efficient and effective.
The LOCOST model thus represents a breakthrough in the field of automatic natural language processing. The work published under the title “LOCOST: State-Space Models for Long Document Abstractive Summarization” was awarded the Best Paper Award at the 18th Conference of the European Chapter of the Association for Computational Linguistics (EACL 2024), held from 17 to 22 March 2024. By offering an efficient and resource-saving alternative to existing models, this work opens up new prospects for text processing, where long documents can be analysed and summarised more quickly and accurately.
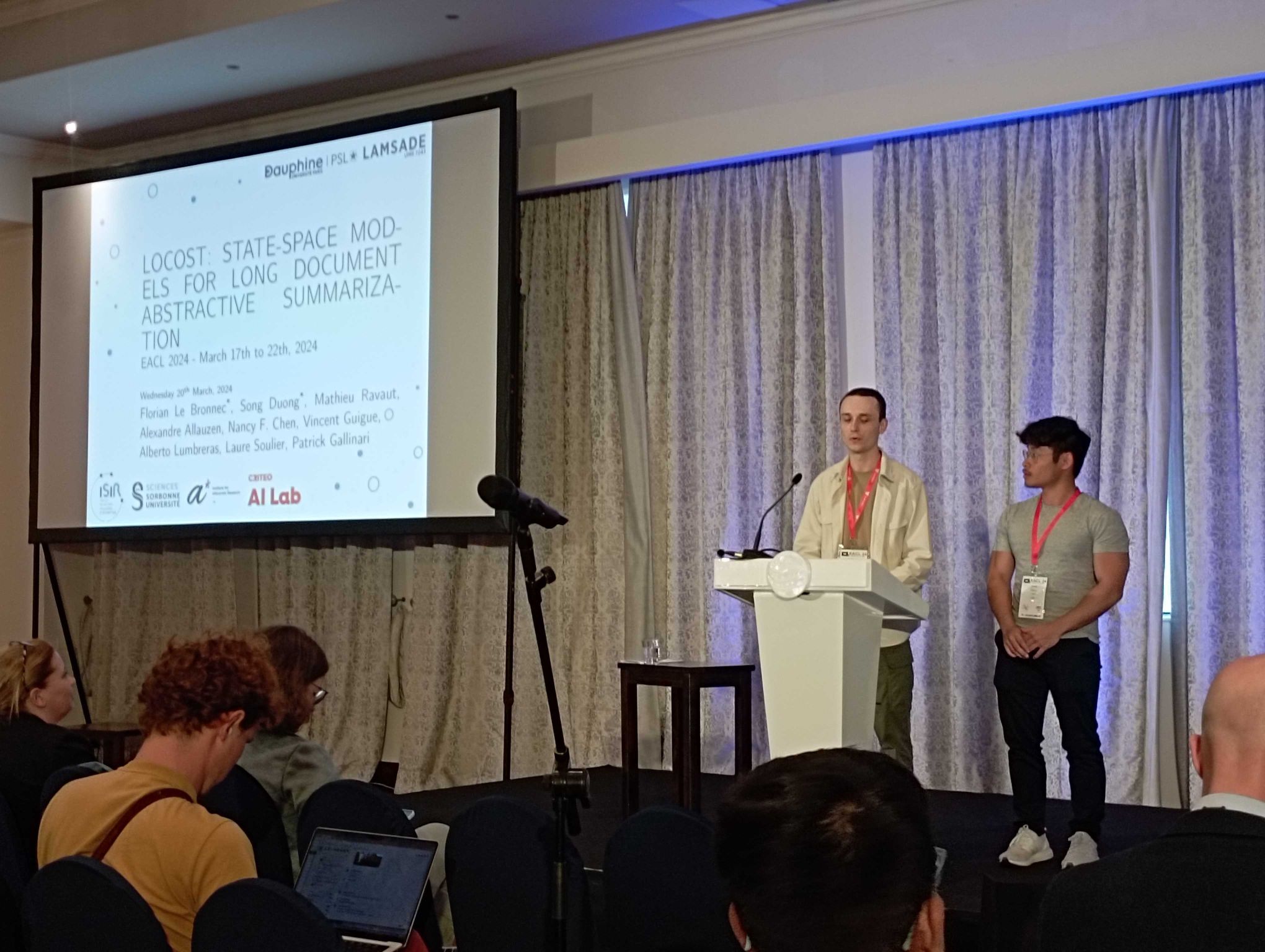
Scientific contact at ISIR: Florian Le Bronnec, PhD student ; Song Duong, PhD student ; Laure Soulier, Senior Lecturer ; et Patrick Gallinari, University Professor.
Link to the paper: https://arxiv.org/abs/2401.17919
Published April 25, 2024.