Project CORSMAL: Collaborative Object Recognition, Shared Manipulation And Learning
In collaborative and assistive robots, handing over objects is a basic and essential capability. Although this capability may appear simple as humans perform hand over flawlessly, it is very difficult to replicate in robots. The handover problem has been investigated in the past and is still an active field of research; it encompasses many subproblems like human intention study, object features extraction, reachability, grasp synthesis and many others.
CORSMAL proposes to develop and validate a new framework for collaborative recognition and manipulation of objects via cooperation with humans able to mimic human capability of learning and adapting across a set of different manipulators, tasks, sensing configurations and environments. The project explores the fusion of multiple sensing modalities (touch, sound, and first/third person vision) to estimate the physical properties of objects accurately and robustly in noisy and potentially ambiguous environments. The main focus is to define learning architectures for multimodal sensory data and for aggregated data from different environments. The goal is to continually improve the adaptability and robustness of the learned models, and to generalize capabilities across tasks and sites. The robustness of the proposed framework will be evaluated with prototype implementations in different environments. Importantly, during the project we will organize two community challenges to favour data sharing and support experiment reproducibility in additional sites.
Context
Cooperation with humans is an important challenge for robotics, as robots will come to work side by side with people. CORSMAL considers a typical cooperative scenario in which a human and a robot hand over an unknown and partially occluded object, such as for example a glass or a cup that is partially filled with liquid, which makes it extremely hard to determine affordances and the appropriate grip points: characterizing partially observable objects is indeed among the most critical issues to instantiate appropriate robot actions.
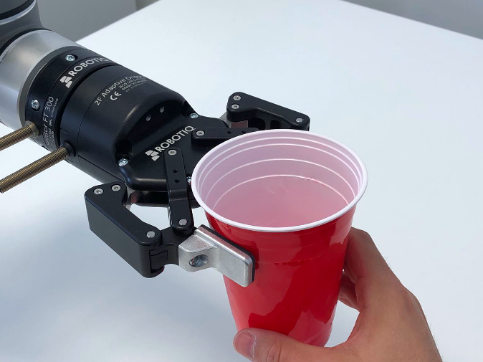
While the mechatronics of robotic manipulators have reached a considerable level of sophistication, the manipulation of objects with unknown physical properties in real-world scenes is still extremely challenging. This is primarily due to a lack of appropriate models and of a sufficient amount of data for training those models. Progress is also hampered by a lack of critical mass of researchers addressing and evaluating the same task using a common dataset.
To address this problem, CORSMAL will build, through the inclusion of physical priors and domain knowledge, learning models that work with fewer data. Moreover, CORSMAL will make available training data and models to other researchers and, to facilitate data sharing and the involvement of the research community, will organize two challenges at international conferences. These challenges and the associated evaluation will illustrate typical human-robot interaction that takes place when the robot is tasked to manipulate arbitrary objects handled daily by humans.
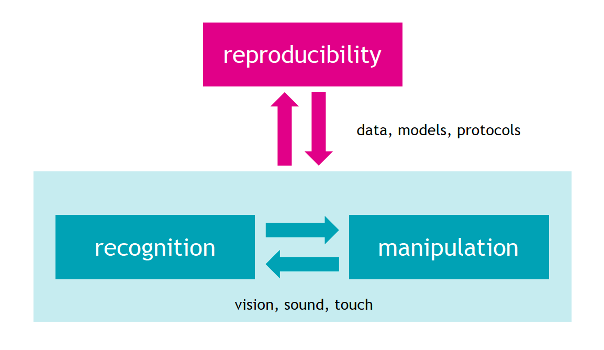
Objectives
The specific scientific questions CORSMAL will address are:
– Can we mimic human capability of learning from few examples and of quickly adapting manipulation by fusing multiple cues?
– Can we replicate human ability to predict affordances and appropriate applicable forces for handover of unknown objects from partial cues?
– Can we efficiently learn trade-offs between manipulator-specific models and generalized models through data sharing for accurate and robust object recognition and manipulation?
To answer these questions, CORSMAL will study, design and validate new learning models and technologies to bridge the gap between perception and manipulation of unknown objects via multimodal sensing, machine learning and model adaptation. CORSMAL aims to create an artificial system capable of learning motion primitives tailored to physical human-robot interaction. The system shall improve its understanding of the environment using learned models and observations of a human to shape robot affordances in object manipulation.
In this project, the ISIR team will focus on the problem of grasping. Indeed, a robot is still not able to grasp an object with unknown properties, in motion and partially hidden, like it is when held in a human hand.
Results
Humans can feel, weigh, and grasp diverse objects, and simultaneously infer their material properties while applying the right amount of force. We want to replicate this with our robotic system. To do so we will need to get information about the interaction between our robot and the object to be grasped.
Our approach will combine the two existing methods to solve the grasping problem: analytical and data driven (illustrated in the figure bellow).
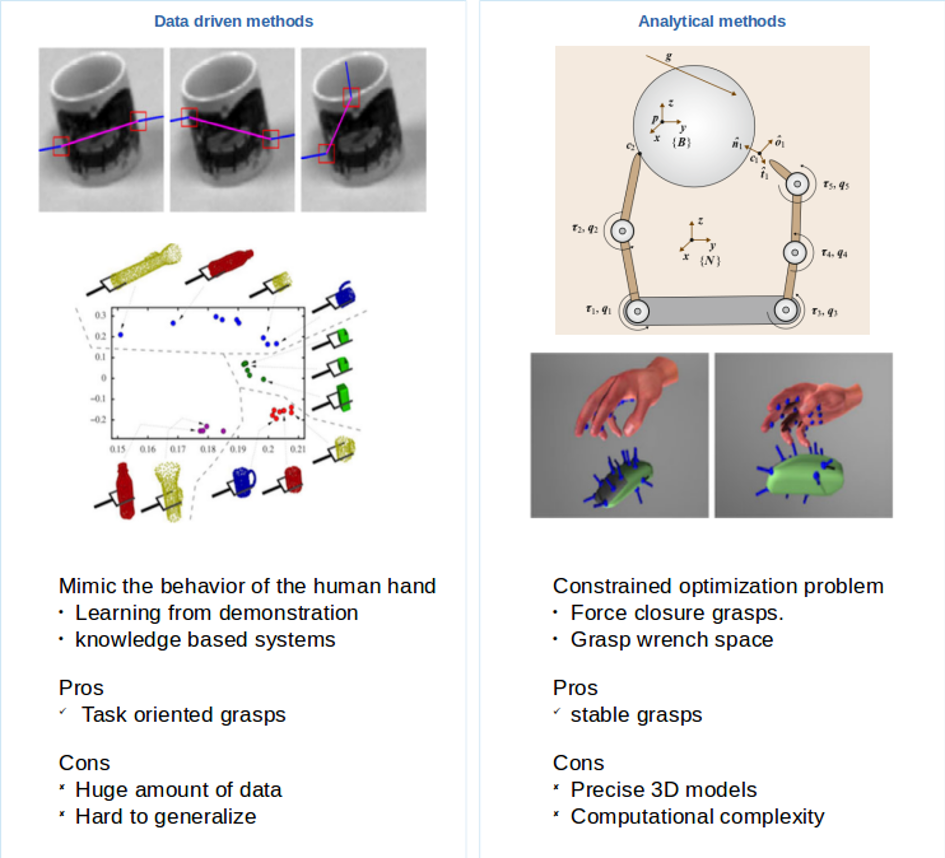
The hand of the robot will be preshaped based on the shape primitive of the object, as inspired by data driven approaches that study how humans perform grasp, allowing to reduce the search space for the appropriate grasps. We will then initiate hand closure until the maximum of contacts is established with the object. Based on the contact points’ locations and joints’ positions, we will obtain the contact locations in 3D space, and the sum of the forces applied to the object. We will perform a search for the applied forces achieving force closure through a combination of contact points. When force closure is not achieved, an analytical approach will help find the contact point where to place/replace a finger.
Our approach will be tested and validated with the Wonik Allegro Hand with 16 DOF equipped with 368 tactile sensors from Xela electronics distributed on the phalanges of the hand, its palm and its fingertips.
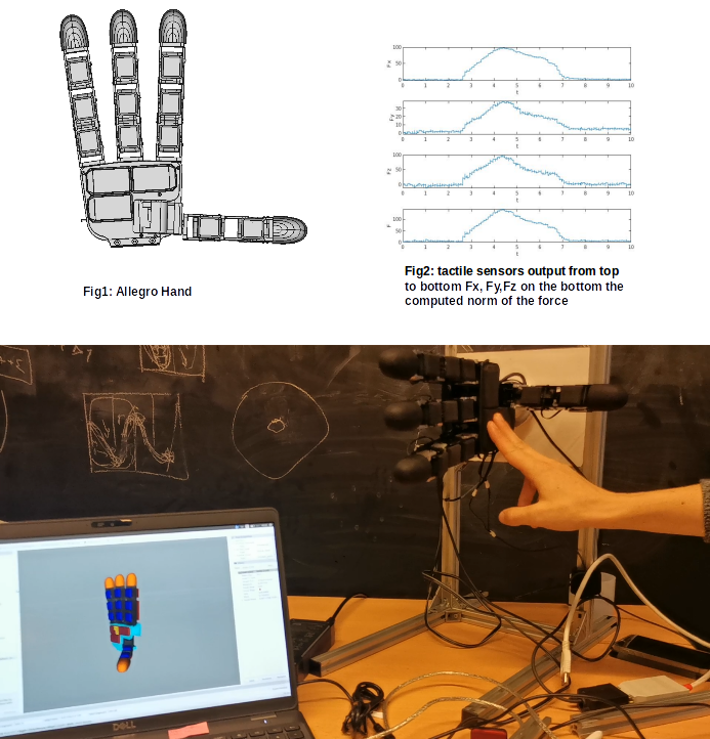
Partnerships and collaborations
This work is supported by the European project CORSMAL under the CHIST-ERA framework, call Topic: Object recognition and manipulation by robots: Data sharing and experiment reproducibility (ORMR) and received funding from the Engineering and Physical Sciences Research Council (EPSRC-UK), Agence Nationale de la Recherche (ANR), and Swiss National Science Foundation.
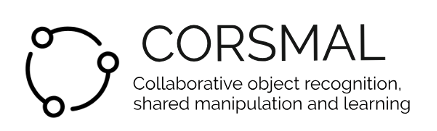
The project partners are:
– Queen Mary University of London – United Kingdom, (project coordinator),
– ISIR of Sorbonne University – France,
– École Polytechnique Fédérale de Lausanne (EPFL) – Swiss.
More about CORSMAL: corsmal.eecs.qmul.ac.uk