RODEO project – Robust deep learning for surgical robotics
The RODEO project is set to reshape surgical robotics by adapting cutting-edge AI. The practical testbed is robotics-guided spine surgery, based on a surgical platform available at ISIR.
In this setup, a 7 DoFs (degrees of freedom) robotic arm is equipped with various sensors (i.e. position, speed, force, electrical conductivity, vibrations) and is used during surgical intervention, e.g., pedicle screw insertion into the spine. This current robotic platform uses a set of already implemented control laws (e.g., position, speed, force control) to execute surgical tasks or subtasks, e.g., drilling a pretrajectory for pedicle screw placement. Before the operation, i.e., “pre-operative”, the patient undergoes a pre-operative 3D CT scan, such that the surgeon defines the medical procedure to follow during the surgery, i.e., “per-operative”.
Although fully automatic controllers can be deployed in safe sub-tasks, surgeons rather rely on the co-manipulation paradigm for sensitive operations, where surgical robots assist medical procedures. In this case, the robotic assistant is expected to faithfully react to the surgeon’s instructions while guaranteeing the safety of both patient and medical staff, and to adapt to the environment.
The context
While the current co-manipulation system [1] is helpful and meets some surgeons’ needs, they could be substantially improved to enhance the surgical experience. The ISIR surgical platform lacks perception and registration modules, and the current procedure assumes that once the patient is positioned for surgery, they does not move and that the spine is rigid. This can make the transfer of pre-operative information complex, imprecise, and dangerous for the patient. Additionally, current controllers fail to represent complex physical phenomena during co-manipulation, such as robot friction (difficult to model because the force applied by the surgeon is unknown beforehand), vibrations (critical for fine-grained manipulation such as peg in a hole), or gravity compensation (crucial for maintaining the robot in a steady position).
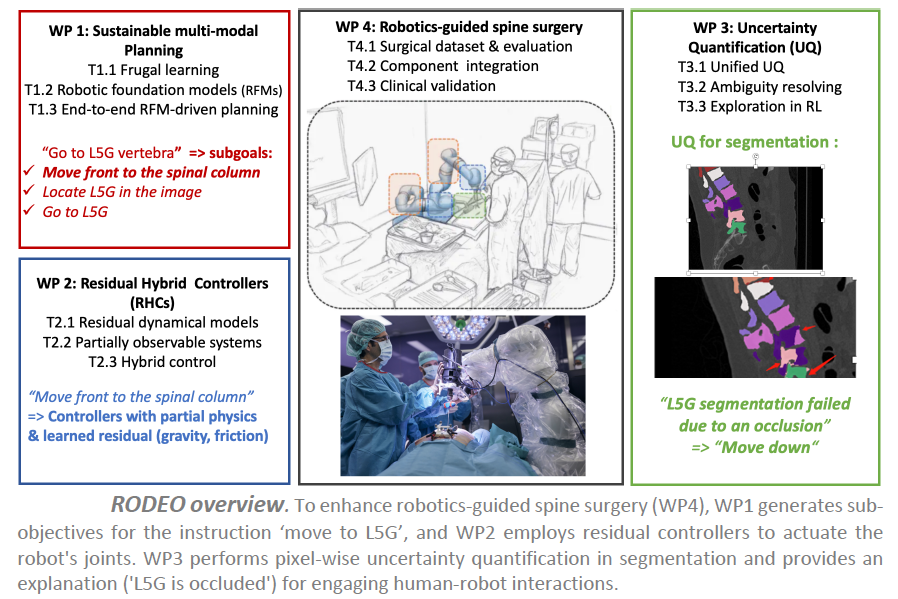
Objectives
RODEO aims to develop the next generation of deep generative AIs, which aims at overcoming the main challenges previously mentioned. The project especially aims at designing AI systems with enhanced robustness in terms of flexibility and reliability while remaining sustainable, and hybrid models able to incorporate physical knowledge of the world.
The central research hypothesis is that these improvements can lead to a major breakthrough in surgical robotics. In general, more reliable AI systems can substantially enhance their acceptance among medical experts and patients, especially by giving to AI systems the opportunity to assess their own confidence or explain their decisions in a human-understandable manner. Hybrid and sustainable models have the potential to substantially improve the level of automation in robot/surgeon co-manipulation. This holds the promise of reducing the cognitive load on surgeons, enabling them to concentrate entirely on medical interventions, ultimately elevating surgical procedures and patient care.
The results
In our spine surgery testbed, we expect major improvement at three main fronts:
– Firstly, we want to design hybrid controllers that can exploit AI to learn the residual components in current controllers that are difficult to model, such as friction, gravitation compensation or vibrations. The goal is to augment the level of automation of some tasks and to further assist in other tasks to release the surgeon’s mental load and enhance the accuracy and the safety of the surgical procedure.
– Secondly, we want to increase the flexibility of the system by using multi-modal deep generative AIs to tackle long-term planning based on language. It will include visual perception by developing AI-based methods for registering pre-operative CT-scans to per-operative depth cameras for enabling robust localization and control, e.g., for breathing compensation.
– Last but not least, we will endow AI systems with the ability to quantify their own confidence, and to explain their decision to the surgical team in an understandable manner, ultimately leading to rich human-robot interactions and dialogs.
Partnership and collaboration
The ANR RODEO project is a single-team project led by ISIR’s MLR (Machine Learning and Robotics) project team, headed by Nicolas Thome, a researcher at ISIR and professor at Sorbonne University.