Project Learn2Grasp: Learning Human-like InteractiveGrasping based on Visual and Haptic Feedback
The Learn2Grasp project’s goal is to develop interactive object grasping strategies for cluttered environments using a robotic arm with a very sensitive dexterous hand. The grasping strategies will be learnt through deep reinforcement learning techniques using simulated and real robots, with data from both RGB-D cameras and tactile sensors. Grasping is a difficult task for learning because it is a sparse reward problem. In order to solve this problem, two approaches will be combined: a bootstrap with human demonstration generated using an immersive teleoperation interface, and an exploration of possible behaviors through advanced quality-diversity algorithms.
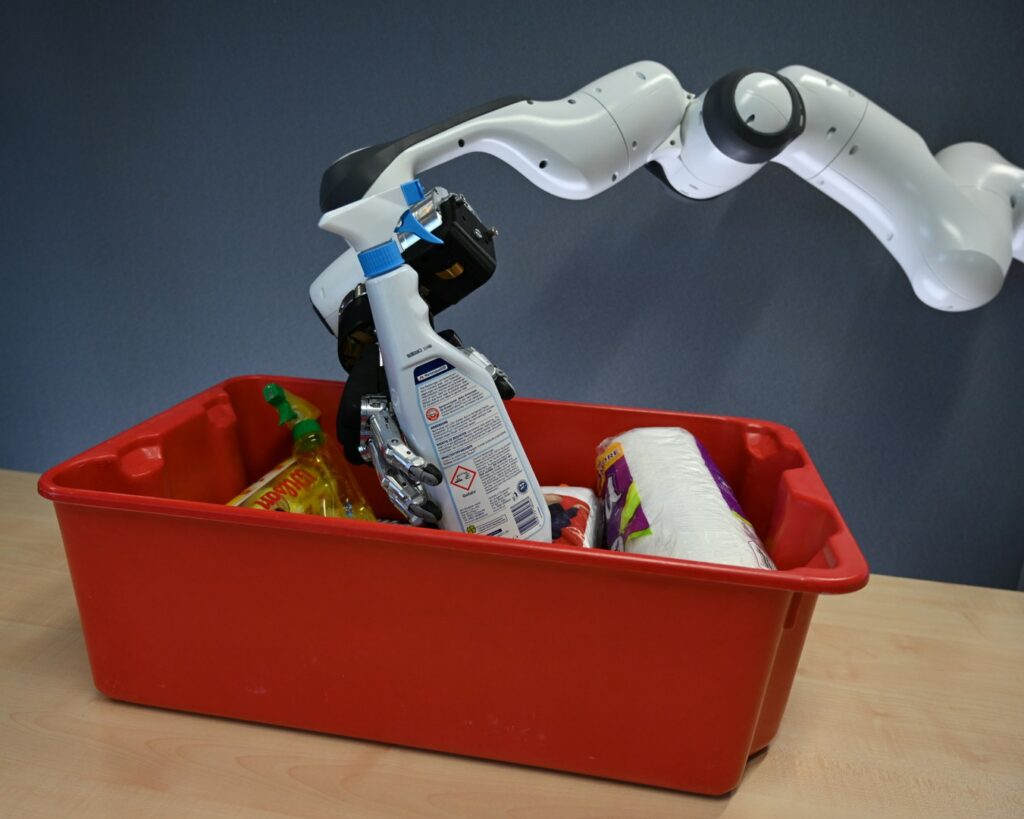
The context
Existing approaches to manipulation based on control theory are efficient but usually require an accurate model of the objects to grasp and their environment. Conversely, machine learning methods can be trained end to end without an elaborate environment mode, but they are often inefficient on hard exploration problems and demand large datasets. A third possibility is learning from human demonstrations, but generalizing beyond the setups from the demonstrations is challenging.
The German-French Learn2Grasp partnership leverages the expertise of two complementary partners to tackle that issue. University of Bonn has a recognized experience in visual perception, scene modeling and learning from demonstration, and Sorbonne University has an expertise in action learning and efficient exploration in sparse reward environments. Both partners will use modern robotic platforms using hands with advanced tactile capabilities.
Objectives
The project’s scientific goals are:
- Combine Bonn’s scene analysis and modeling techniques with Sorbonne University’s diverse policies generation methods, in order to develop robust, efficient and data-efficient grasping motion generator.
- Integrate the tactile modality with vision-based perception in order to learn more flexible and robust closed loop policies.
- Learn from hybrid datasets and optimally exploit data from simulation, human demonstrations (through a teleoperation interface) and real robot experiments.
Those tasks are structured in 4 work packages:
- WP1: Structured Multimodal Scene Modeling and Prediction (Leader: University of Bonn),
- WP2: Learning Interactive Grasping (Leader: Sorbonne University),
- WP3: Technical Integration (Leader: University of Bonn),
- WP4: Management and Scientific Collaboration Oversight (Leader: Sorbonne University).
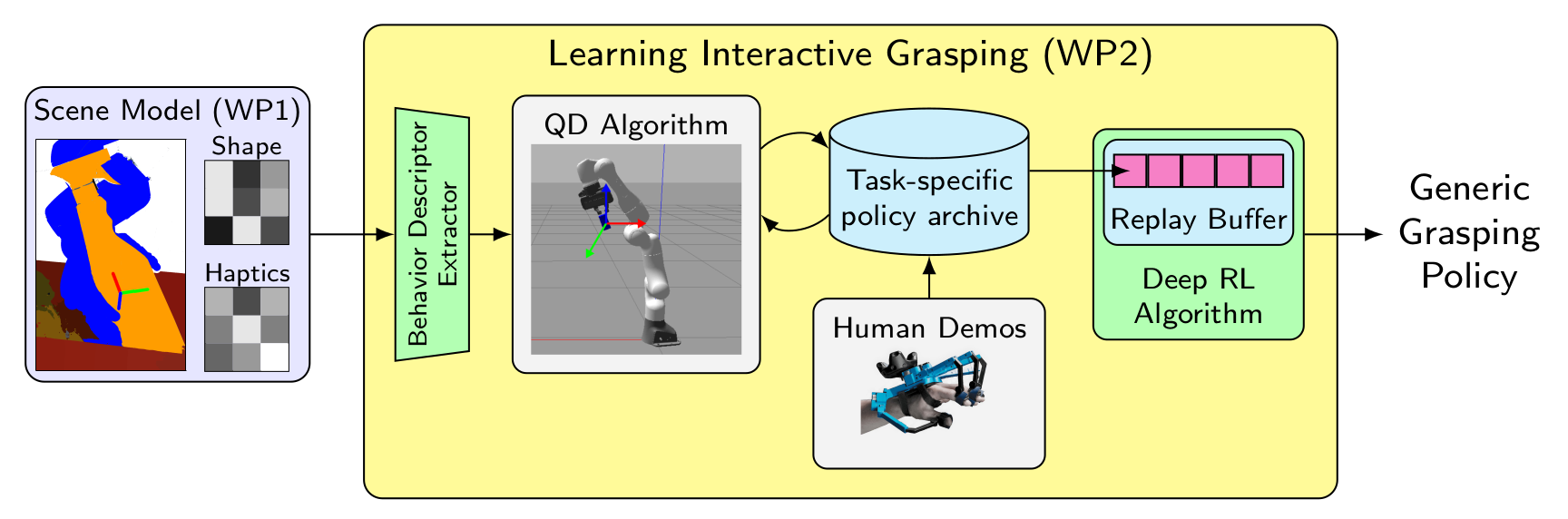
The results
The project’s outcome will be the realization of an integrated system using the developed visual and tactile perception capabilities to build scene representations, and using those representations to efficiently learn, with limited datasets, diverse open-loop and closed-loop policies allowing a robust grasping in cluttered environment. This system will be evaluated on simulated and real environments.
Another expected outcome of Learn2Grasp is to build lasting scientific collaboration links between AIT team at University of Bonn and ISIR at Sorbonne University, in order to develop a centre of expertise on learning for manipulation, covering all aspects from perception and representation learning to action generation.
Partnerships and collaborations
Learn2Grasp (ref. ANR-21-FAI1-0004) is a research collaboration between:
- AIS team at University of Bonn,
- and ISIR at Sorbonne University.
This collaboration is realized in the framework of the 2021 bilateral German-French program for AI research (MESRI-BMBF). It will last 4 years, from 2021 to 2025.
The project is led at Sorbonne University by Miranda Coninx, associate professor in ISIR AMAC team, and at University of Bonn by Prof. Dr. Sven Behnke, head of AIS team (Autonomous Intelligent Systems).