Cooperation of multiple reinforcement learning systems
Vertebrates are able to learn to modify their behavior based on rewards and punishments. This learning, called “reinforcement learning”, is also the subject of much research in Artificial Intelligence to increase the decision-making autonomy of robots.
How to learn by rewards and punishments, as fast as possible for a minimal computational cost? This is the question we are addressing by combining reinforcement learning algorithms with complementary characteristics.
This interdisciplinary project aims to improve the performance of robots, but also to better explain learning in vertebrates.
Context
Reinforcement learning distinguishes two main families of algorithms:
– those who build a model of the world and reason on it to decide what to do (MB or model-based). They require a lot of computation to make a decision, but are very efficient, learn to solve a problem with few trials and relearn just as quickly if the task changes.
– those without models that learn from simple state-action associations (MF or model-free). They are very cheap in computation, but on the other hand learn slowly and relearn even less quickly.
Vertebrates, on the other hand, are able to exhibit goal-directed behavior resulting from deductions about the structure of the environment. With prolonged learning, they develop habits that are difficult to challenge. It has been widely accepted since the mid-2000s (Daw et al., 2005) that MB algorithms are a good model of goal-directed behavior, and MF algorithms a good model of habit formation.
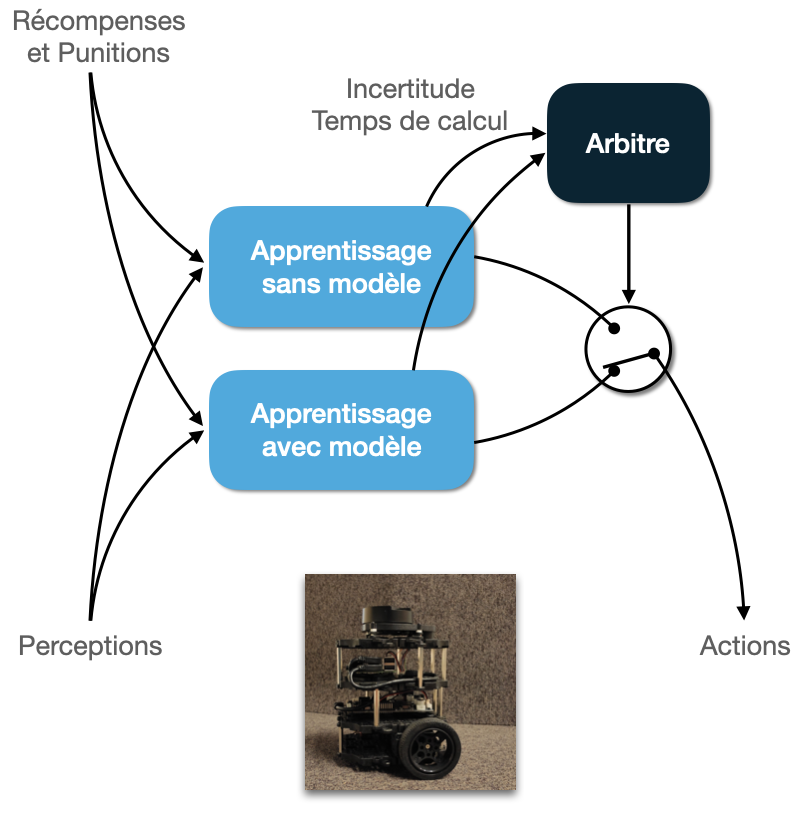
Objectives
From a robotics and AI point of view :
We aim at defining methods to coordinate these two types of algorithms in order to combine them in the best possible way, to learn quickly and to adapt to changes, while minimizing computation when possible. We test our realizations in robotic navigation and human-machine cooperation tasks.
From a neuroscientific point of view :
We rather seek to explain the observed interactions between flexible and habitual behavior, which do not necessarily seem optimal. This implies that the coordination methods developed for robotics and for neuroscience are not necessarily identical.
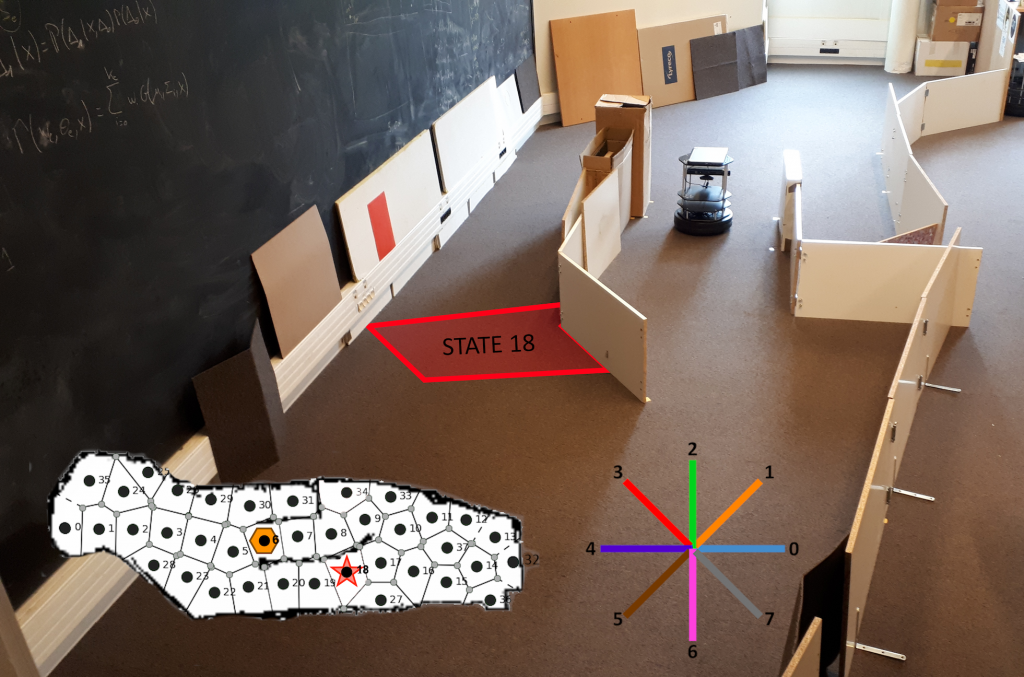
Results
We initially proposed a method for coordinating MB-MF algorithms to explain competition and cooperation effects between learning systems in rats (Dollé et al., 2010, 2018).
It was then adapted for use in robotic navigation (Caluwaerts et al., 2012), and equipped for the occasion with a context detection system to learn and re-learn quickly when the task changes. The development of a new coordination criterion explicitly taking into account computation time has allowed to propose a new robotic system with maximum performance, identical to that of an MB algorithm, for a computational cost divided by three (Dromnelle et al., 2020a, 2020b).
In parallel, models have been developed to explain decisions and response times in humans (Viejo et al., 2015) and macaques (Viejo et al., 2018).
The overall achievements of this substantive project have been summarized in the paper Adaptive coordination of multiple learning strategies in brains and robots (Khamassi, 2020).
Partnerships and collaborations
This work has been carried out in the framework of various projects, financed by the ANR (LU2, STGT, RoboErgoSum), the City of Paris (Emergence(s) HABOT), the B2V Memory Observatory, the CNRS, etc.
They rely on collaborations with:
– Andrea Brovelli, Institut de Neurosciences de la Timone (INT) of Marseille, in France,
– Raja Chatila, Benoît Girard and Mehdi Khamassi, Institut des Systèmes Intelligents et de Robotique (ISIR) of Paris, in France,
– Rachid Alami and Aurélie Clodic, Laboratoire d’analyse et d’architecture des systèmes (LAAS) of Toulouse, in France,
– Emmanuel Procyk, Stem cell and Brain Research Institute (SBRI) of Lyon, in France.